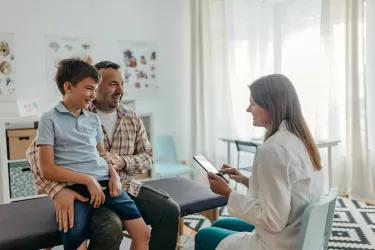
Health & Medicine
Artificial intelligence is changing the way your GP works
As AI technology advances, understanding and integrating the perspectives of older adults can lead to more user-centred and effective AI solutions in healthcare
Published 5 December 2024
In the era of artificial intelligence (AI), medical AI is gaining significant attention for its potential to revolutionise healthcare and enhance a wide range of medical services.
In recent years, AI-assisted disease diagnosis and prevention have begun to specifically target age-related conditions like Alzheimer's disease.
These tools include a deep-learning algorithm using voice and facial expressions to identify patients with early-stage Parkinson’s disease and AI tools designed to detect Alzheimer's disease from brain images.
Despite these advances, there has been little focus on ageing in the general development of medical AI technologies.
Specifically, we lack an understanding of how older adults are represented in training AI datasets and design processes. If this is not addressed, AI is prone to reinforcing bias, which can lead to misdiagnoses and a lack of generalisation to the wider population.
Questions about the acceptance and adoption of these technologies also persist.
So as our population ages, it becomes more important to understand how older adults perceive medical AI technologies and whether they are willing to embrace AI-assisted disease diagnosis and other healthcare services.
Health & Medicine
Artificial intelligence is changing the way your GP works
Issues with data representation and bias are longstanding challenges in developing medical AI models. Older adults are often poorly represented in the construction of data sets, apart from those exclusively targeted to age-related diseases.
For example, research shows that because AI training datasets are focused on young and healthy individuals, automated algorithms frequently miss or misinterpret sleep disorders in older patients.
Developing representative datasets for older adults is challenging due to difficulties in accessing the specific types of data needed while ensuring diversity in gender, ethnicity and age.
Curating such intricate datasets can take years, leading to the underrepresentation of older patients unless the focus is explicitly on geriatric diseases.
Even when research prioritises data from the older population, concerns about data quality persist.
In ophthalmology, for example, cataracts are a common age-related eye condition where the eye’s lens becomes cloudy, leading to blurred vision and in advanced cases, vision loss.
This cloudiness can make it more difficult to capture high-quality retinal images in older adults for AI training.
Additionally, certain patient groups may face limited access to healthcare services, making it challenging to obtain their eye image data. This lack of representation can lead to AI models that perform poorly in rural and remote areas.
Enhancing data diversity and accessibility is crucial to ensure that AI implementations serve all populations effectively.
Health & Medicine
Explaining AI’s role in precision medicine
It is also important to recognise the general lack of consumer research on how older adults perceive AI technologies in healthcare.
It can be challenging to gather opinions from relatively marginalised older communities, especially those with restricted socio-economic status, lower levels of digital literacy, and limited access to medical resources.
For example, in a recent survey conducted by the Centre for Eye Research Australia, due to accessibility issues, the respondents were predominantly from higher socio-economic backgrounds and possessed higher levels of education.
While many participants expressed openness to medical AI, the results may be biased due to the demographics of the sample.
The acceptance of medical AI is also often viewed as being primarily reliant on the end-users, who are the patients. However, acceptance is a more complex issue that also involves clinicians, whose attitudes can ultimately influence whether patients accept these technologies.
For instance, in the context of age-related eye diseases, it is crucial to distinguish between two types of clinicians.
The first group consists of eye care professionals, including optometrists and ophthalmologists, who typically have access to specialised technologies in their clinical settings. These professionals can easily obtain high-quality fundus images (the back surface of the eye) and, in some cases, have established collaborations with industry partners.
For example, some optometry stores use AI to assist in diagnosing conditions like glaucoma, particularly in difficult cases.
Health & Medicine
Overcoming our psychological barriers to embracing AI
In contrast, non-eyecare professionals like general practitioners (GPs), face different challenges. Patients typically do not visit optometrists or ophthalmologists unless they experience symptoms, making GPs crucial in the early diagnosis of eye diseases.
However, due to a lack of specialized knowledge, GPs may feel less confident in identifying eye diseases in their patients. In such cases, AI could be a valuable tool in helping GPs detect referable eye diseases, like diabetic retinopathy and refer patients to the appropriate eyecare professionals.
Nonetheless, the extent to which GPs accept AI-assisted diagnosis in this context remains uncertain.
Some AI-assisted diagnostic models are more easily accessed by older patients. In these cases, AI explainability is directly provided for the end user, which may improve user experience and acceptability.
For instance, health apps with embedded medical AI models for skin lesion assessment (dermatology) are already available on the market providing a preliminary diagnosis based on photos of the skin taken by a mobile camera.
Depending on the capabilities of AI models in the apps, AI explainability can range from highlighting key areas in the skin image to identifying the most significant contributing factor, like recent sun exposure.
However, in fields like neurology and ophthalmology, AI-assisted diagnosis typically requires mediation by clinicians.
These professionals interpret the AI-generated report and based on their analysis, communicate the diagnosis or treatment plan to patients.
Arts & Culture
The music app supporting dementia care at home
Here, AI explainability is geared toward clinicians rather than the patients themselves as the reports are often dense with medical terminology – reflecting the nature of the training data used to develop these models.
In the future, truly explainable models would become interactive by providing outputs, similar to conversational AI systems like ChatGPT, allowing users to ask follow-up questions.
The model would then respond to those questions, fostering a more dynamic understanding of the results. These models could help laypeople, including older patients, better comprehend the diagnostic outcomes.
Several new questions have emerged around medical AI and aging populations. The first relates to enhancing interdisciplinary collaboration.
While collaborations between AI scientists and medical professionals are increasingly common, integrating the insights and theories of social scientists remains challenging.
Addressing this requires dismantling existing barriers to foster meaningful engagement and ensuring the human factor is adequately considered in the development of medical AI technologies.
But this is also a two-way process that will involve enhancing social scientists' understanding of technological advancements, enabling them to effectively contribute to the research process.
Health & Medicine
Using AI to predict hospital costs in real time
The second critical question revolves around the agency of older adults in the adoption and development of medical AI technologies.
Traditionally viewed as passive recipients of technology, it is becoming increasingly important to empower older patients by giving them a more substantial voice in shaping the future of medical AI.
As technology advances, understanding and integrating the perspectives of older adults can lead to more user-centred and effective AI solutions in healthcare.
This shift not only acknowledges the valuable insights that older adults can provide, but also ensures that medical AI technologies are responsive to their specific needs and preferences.