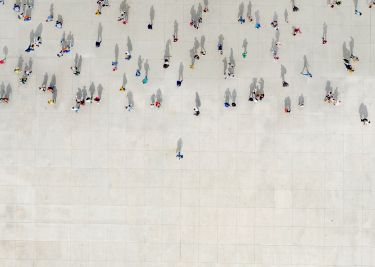
Health & Medicine
How data can help prevent overdiagnosis
Adding Artificial Intelligence research to precision medicine is the future – but any collaboration between AI researchers and clinicians must include the human factor
Published 23 February 2022
Artificial intelligence (AI) research in medicine is evolving – promising faster, larger-scale and new solutions to complex health problems.
It’s a technology that has become increasingly prevalent in medicine – particularly, in the field of ‘personalised’ or ‘precision medicine’, which aims to develop treatment plans for patients that increases individual treatment effectiveness while also decreasing negative side effects.
This focus on a patient-specific approach is growing in all areas of medicine, in particular in oncology, and is now accepted as ‘best-practice’ by healthcare workers and government bodies, as well as patients.
But a personalised medicine approach is complex and is still far from the standard of care. However, with the help of AI, the reality seems closer than ever.
So, what’s standing in the way of AI research when it comes to its application in the area of medicine?
Health & Medicine
How data can help prevent overdiagnosis
AI calculations are most helpful whenever ‘big’ or complex data is generated and involves higher computational power that goes far beyond the needs of a simple data repository.
But, AI research in medicine is still in its infancy and the reality is that few AI applications have led to ground-breaking changes in patient care despite the increasing number of FDA-approved AI software packages in the US.
In common with traditional clinical research, fundamental principles apply to AI research – it needs to earn trust by building credibility which requires applying a robust, validated and reproducible methodology.
Erroneous or overly complex methodologies, a lack of ‘explainability’, a biased study design or cohort selection can lead to non-reproducibility of results and this can cause roadblocks and mistrust in any AI research discovery.
AI research in medicine falls under the umbrella of ‘translational research’, which aims to combine fundamental science discoveries with clinical findings to generate novel treatment approaches that aim to improve healthcare.
Examples of translational research include discoveries like lab-designed targeted compounds – like immunotherapies commonly used in oncology – but it is increasingly playing a role in other medical fields, like infectious and chronic diseases.
Health & Medicine
Using AI to predict hospital costs in real time
Traditional clinical research is commonly led by clinical healthcare workers and aims to improve outcomes for trial patients or change the management of future patients.
These clinicians use evidence-based knowledge to improve the management of patients. They are trained to adapt and think outside the box. But many adaptive approaches are forced upon them by special patient needs and often don’t change their fundamental approach.
AI operates differently.
This research is often led by non-clinical groups – like physicists, bioinformaticians, bioengineers and data scientists. AI scientists are experts in calculating algorithms using complex mathematical formulas and these specialised skills are constantly challenged by rapidly changing developments.
But some of these AI algorithms may never end up in clinical practice because the AI methodology and results are challenging to explain to clinicians and, as a result, may be mistrusted and sometimes rejected.
In a collaboration between the University of Melbourne, Peter MacCallum Cancer Centre, Royal Melbourne Hospital and Walter Eliza Hall Institute, Melbourne, our research in the emerging field of radiomics in pancreatic cancer is promising to change the way oncologists treat the disease from diagnosis.
Health & Medicine
Would you trust AI with your mental health?
But there’s a lot of work to do.
By looking at research that’s been published over the last years we can get an idea of the amount of theoretical and discovery work that goes on to become a medical reality.
The number of publications in the National Library of Medicine’s bibliographic database, Medline, on AI has increased exponentially over the last three decades – starting out with 11 publications in 1981 to over 11,000 publications in 2018.
Just two years later, in 2020, that figure had ballooned to 21,000 publications.
This correlates to a doubling of numbers over the last three years and an impressive 1972-fold increase over the last 30 years (see graphic below).
However, each discovery also encounters setbacks.
This pattern is shown in the field of ‘AI’ and AI and Medical Imaging’ with two periods of a 15 to 35 per cent decline in the number of Medline publications compared to a former peak year.
AI research may promise to answer pertinent clinical questions but, so far, few have been able to deliver. And this goes back to the increasing number of non-reproducible results, which AI radiomics research groups are recognising as a pitfall of AI research methodology in medicine.
Health & Medicine
The simple, ethical case for gene editing
Increasingly, there’s acknowledgement in the AI field that dealing with people and their innumerable human factors aren’t easily integrated into an algorithm.
Plugging an AI app into the world of clinical practice isn’t a simple software extension or an add-on – it needs a new collaborative approach.
AI scientists, like clinicians, need to create a common platform of understanding to come up with a robust and validated methodology and produce meaningful results,
Communication among multidisciplinary team members and sustainable collaboration is the key to success in both virtual and non-virtual worlds.
This collaboration will make the difference between research results that will lead to better patient outcomes and those that will never be implemented in practice.
Clinicians have to be able to clearly communicate their personal and professional experience, including ‘intuition’ or ‘gut-feeling’. AI scientists meanwhile must understand which clinical factors are important to develop a methodology that will be applicable to a wider population.
But the ultimate goal of both should always be to improve patient care and this focus mustn’t get lost amid the current AI hype.
Every AI adventure could lead to a wonderful discovery, and data cannot physically harm a human being. However, when science is misinterpreted or abused it can be misleading to the point of being destructive. So, AI research in medicine must be scrutinised rigorously because the public expects error margins of close to if not zero.
Openness, transparency and the willingness to learn from and effectively communicate with each other will most certainly lead to new discoveries that benefit patients.
Banner: Getty Images
We acknowledge Aboriginal and Torres Strait Islander people as the Traditional Owners of the unceded lands on which we work, learn and live. We pay respect to Elders past, present and future, and acknowledge the importance of Indigenous knowledge in the Academy.
Read about our Indigenous priorities