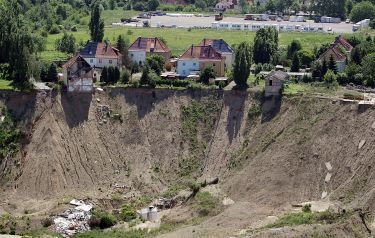
Sciences & Technology
Decoding data to predict landslides
A new data-driven tool uses satellites to accurately predict devastating natural disasters before they occur – locating potential land failures to protect at-risk communities
Published 4 February 2022
Landslides are devastating and often unpredictable.
In 2017, without warning, a catastrophic landslide hit Xinmo Village in Southwest China, engulfing homes in an avalanche of rock and mud and burying dozens of people alive.
On the other side of the world, Mount Stromboli, a volcano in the Tyrrhenian Sea off the northern coast of Sicily, collapsed several times in 2015 and 2016, causing massive rockfall and destruction in the surrounding areas.
Sciences & Technology
Decoding data to predict landslides
But what do these two unrelated natural events have in common?
University of Melbourne researchers have discovered that these two events could have been predicted using freely available space satellite data and a new data-driven mathematical prediction model.
Satellite radar images have long been used to predict and capture the early warning signs of impending natural disasters, including landslides – tonnes of rock, earth, mud or debris moving down a slope, often destroying everything in their paths.
In January 2022 alone, there have been several notable natural disasters – from the tsunami in Tonga to the dramatic rockfall in Brazil – both causing great loss of human life and taking a toll on the environment.
But with enormous areas of high-risk slopes to monitor, the challenge for tracking satellite data has been where to focus the analysis.
That is, until now.
In a new study, University of Melbourne researchers have developed a data-driven prediction method that provides better forecasting when it comes to the location and geometry of impending landslides.
Sciences & Technology
Sensing a serious problem
Using machine learning to identify the most common and unique characteristics of landslides captured from satellite data, the team has built a new prediction model that can accurately identify the location of impending failures well before they occur.
Professor Antoinette Tordesillas from the School of Mathematics and Statistics and the study’s lead investigator says the intention behind this technology is to prevent loss of life by mitigating the risk of disasters – like some of the recent land failures we’ve seen around the world.
“This new model has been designed to be used with satellite data – which is free and widely accessible,” says Professor Tordesillas.
“Our model opens the door for the first truly accessible tool that works for vast area coverage including remote inaccessible areas using data that is free and not reliant on expensive industry technology.”
This model builds on earlier work by Professor Tordesillas and colleagues that relied on high-resolution radar technology to detect slope movement, which is useful for mining companies but not feasible for at-risk communities or for covering large areas.
“Earlier work gave accurate early warning on the location and timing of failure for a single slope. But if your data covers a huge area and the target location is very small then you get severely imbalanced data.
Sciences & Technology
Using maths to map mines deep underground
“This is a big problem because the signal from an impending failure is then drowned out from the vast amount of information, resulting in degraded forecasting performance.
“We have fixed this problem.”
Pinpointing the location of an impending land failure – in other words, landslides – from space has historically been a challenging task.
By using this newly discovered information on rock failure and applied mathematics, the present model can identify the location of the imminent land failure, providing early warning to communities at risk.
“Our approach provides important intervention and timely risk assessment from satellite data, especially for remote areas that are difficult, if not impossible, to access. We do this by extracting space and time patterns of evolution of ground displacement,” says Professor Tordesillas.
If the satellite captures early signs of an impending failure, then the team knows how to extract information efficiently from the (invariably large) amount of data.
This tool can literally pinpoint the impending failure of a location, even if the area is tiny – or less than one per cent – of the whole area that’s being monitored.
Sciences & Technology
Using maths to prove computer security
This kind of early warning is crucial to alert and evacuate communities, as well as safeguard surrounding areas from potentially catastrophic risks.
Just like the case of the avalanche in Xinmo Village and the rockfall in Brazil, with early warning and intervention, the community could have been evacuated – potentially saving many lives.
By using satellite data, the tool can be used anywhere in the world, particularly in countries that are at higher risk of landslides, like Nepal and Italy.
Helicopter surveys of the unreachable site in Stromboli Volcano, where the 2015-16 rockfalls took place, helped prove the tool can predict rockfalls from space with pinpoint accuracy.
The technology has also been supported by industry, government, and academic partners worldwide, including Ground Probe, a member of the Orica Group.
UNESCO Chair for Prevention and sustainable management of geo-hydrological hazards, Professor Filippo Catani said:
Sciences & Technology
The algorithms of art
“This new method leverages space-time multivariate analysis to sift through huge radar satellites datasets in search of impending disaster at both global and local scale. This may offer a new component of practical early warning systems using satellite data, capable of anticipating the occurrence of landslides, sinkholes, and slope failure-related disasters “
The predictions from this model can also be used in the continued monitoring and management of future climate change-induced geohazards – like landslides and avalanches which can trigger cataclysmic tsunamis, as we’ve seen this year, says Professor Tordesillas.
“With rising sea levels, temperatures and extreme rainfall events, it is likely we will see many more landslides and related natural disasters. This technology will continue to be critical in protecting at-risk villages, cities and communities through early warning.”
Banner: Getty Images