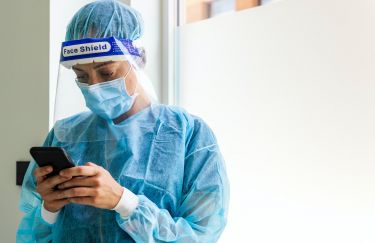
Health & Medicine
Gathering lessons from COVID-19 health workers as they happen
Large-scale testing is key to controlling COVID-19 and statistical modelling shows that analysing grouped samples can give a better and more efficient indication of the prevalence of disease
Published 2 September 2020
Australia’s Victoria state, which went into strict Stage 4 restrictions in July after COVID-19 cases surged, is on a testing blitz to identify every positive case possible, in the hope of quickly controlling the outbreak.
Currently, they are averaging around 20,000 tests every day, with most results coming back within three days.
But is that enough?
There are calls for mass testing of asymptomatic people to get a full picture of how the disease is moving through the community. This could be an important step in controlling outbreaks, but how can we massively increase our testing without overwhelming the testing laboratories?
There is a simple answer – group testing. By combining samples from multiple people into a single test, 20,000 analyses could cover 100,000 people. Further analysis would be needed to narrow down the positives found in group tests, but it would mean that 20,000 tests could be analysed in 6 to 12 hours instead of 48 to 72.
Health & Medicine
Gathering lessons from COVID-19 health workers as they happen
Professor Aurore Delaigle is a professor of statistics at the University of Melbourne who develops statistical models of disease incidence. She says that we should add group testing to our monitoring programs so that more people can be tested more quickly, and the true community incidence of COVID-19 can be determined.
This call for group, or pooled testing is being made around the world and has already been used in places like China and the US. In China, group testing was used to test 9 million residents of Wuhan in only 10 days.
“Suppose that we can only process 1000 tests each day,” says Professor Delaigle.
“If there is a 1 percent prevalence, then in 1000 individuals, on average, only 10 will have the disease.”
“But if you run 1000 tests, and in each test you combine the samples of 10 individuals, then you capture 10,000 individuals. Instead of catching 10 people that are sick, on average you will catch 100 of them. So you can learn a lot more.”
Professor Delaigle says that to accurately determine the prevalence of the disease, you can’t just test a lot of people. You also need to know who you are testing.
In Victoria, positive cases are rapidly dropping, but at the peak of the outbreak around 2 percent of the 20,000 plus daily tests were positive.
Sciences & Technology
Test smarter not harder for an agile system
However, this doesn’t mean that 2 percent of Victorians had been newly infected with COVID-19, because in most cases, people who get tested are those either exhibiting symptoms, close contacts of positive cases, or live or work in identified clusters or ‘hot zones’. So people being tested aren’t necessarily representative of the whole population.
This seems appropriate under the circumstances, since the government wants to pick up as many cases as possible so that those infected and their close contacts can be safely quarantined. With limited resources, the best way to find an infection is to test those most likely to be infected.
But we also know that many people infected with COVID-19 show no symptoms at all. And in regional Victoria and other states with no or very low known cases, a broader, large-scale testing regime across the whole community may be a useful way to catch new cases early, rather than just testing those deemed ‘at risk’.
“There are various ways to choose people randomly in the population,” says Professor Delaigle.
“Sometimes one may want to focus on some regions or groups more than others and in that case, to get good estimators of the prevalence, we make adjustments to take the sampling numbers of each group into account, which isn’t always straightforward.
“Regardless of the sampling strategy, by pooling individuals together in small groups and testing only the groups, we can take more people into account and increase the precision of estimators.”
Politics & Society
How can we open up Melbourne while tackling disadvantage?
By running grouped data through statistical models, Professor Delaigle and her colleagues can calculate not just the overall incidence, but the incidence by age, sex, location, or any other factor of interest, providing they have the information to fully understand the data.
Professor Delaigle points out that there is always some information you need that is missing. And when this missing information isn’t completely at random, a correction factor needs to be applied.
“Often, people don’t show up for testing for a reason, and if the reason is associated with what you want to measure, the disease in this case, you have to take into account that missingness.
“For example, suppose that the sick individuals or aged people refuse to get tested. If you don’t take that into account then your results are going to be wrong – you’ll have a biased the sample.
“However, there is a correcting factor that can account for the reason why they didn’t come. So you need to model the missingness and you include that when you estimate your prevalence.”
Professor Delaigle says that we also bias the analysis when there are false positives and false negatives. Once you know the probability of a false positive and a false negative, you need to apply correction factors to get accurate estimators of the prevalence. Professor Delaigle has developed some techniques for doing this for grouped data.
There could also be dilution issues – by combining samples you risk diluting the test so much that you miss positive cases. Professor Delaigle has worked on ways to combat this by including some correction factors for dilution in the models.
Health & Medicine
Dealing with COVID-19 in aged care
Finally, the prevalence evolves every day and there are also ways to combine older data with newer data and update the estimator of prevalence as it evolves over time.
While grouping allows testing to capture many more people, the downside is you don’t know which individuals in a particular grouped sample have the disease. But Professor Delaigle says this is easily solved by retesting the individuals from just the positive samples. When overall incidence is low, as it is for COVID-19, this still allows many more people to be tested more quickly compared to testing every individual sample.
The government could also run a hybrid testing regime, where some high-risk people are tested individually, in parallel with a group-testing program that tests people in the community more randomly.
As we move from a mass reduction strategy to a more controlled ‘suppression’ strategy, Professor Delaigle says now is the time to introduce mass grouped testing.
“The more people we test, the quicker we can identify outbreak clusters and the quicker we can move to control them.”
Banner: Getty Images