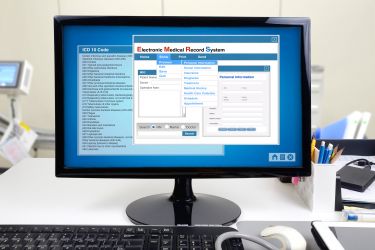
Health & Medicine
The dark side of electronic medical records
The increasing use of digital technologies in medical screening may lead to a rise in overdiagnosis that could be prevented by a new focus on patient data
Published 22 January 2022
Can knowing too much harm us? When it comes to medicine, in some cases, yes, absolutely.
Finding and treating diseases early saves lives and reduces health costs. It is why health systems have been increasingly undertaking proactive screening – administering clinical tests on individuals who don’t have any symptoms but may be at risk. Examples include screening for diseases like bowel cancer or breast cancer.
The ubiquity of digital technologies in our lives has the potential to take proactive screening to a new level – digital screening. This involves using information collected by our smartphones, smartwatches, keyboards, social networks, and wearable technology and analysing it using artificial intelligence algorithms to identify at-risk individuals.
For example, the early onset of Parkinson’s Disease could be identified from the changing patterns of how someone types, while the risk of heart problems can be identified from abnormal heart rhythms detected by a smartwatch.
Health & Medicine
The dark side of electronic medical records
While all this may sound good, the problem is that proactive screening can find things that might not be worth finding. There is overwhelming evidence that some diagnostic tests used in screening can find abnormalities that, while meeting the current definition of a disease, might have never made some people sick.
These ‘diseases’ that would have never generated any problems are what we call cases of overdiagnoses and can lead to unnecessary and sometimes harmful treatments. And the emergence of digital screening could make this problem worse.
Overdiagnosis as a phenomenon has been seen in many well-known diseases, from some common cancers to attention deficit disorder. The most common scenario of overdiagnosis is when we actively seek diseases in asymptomatic patients, especially among healthier segments of the population.
For example in some countries screening for thyroid cancer has led to a two-fold increase in the incidence of thyroid cancer, but no change in mortality. In other words, the screening hasn’t reduced deaths.
Similarly, studies have shown that screening for Attention-Deficit/Hyperactivity Disorder in children can lead to simply the youngest children in a school class being diagnosed.
Overdiagnosis has been observed for many cancers, including prostate cancer and breast cancer, as well as conditions like chronic kidney disease, gestational diabetes, high blood pressure and autism spectrum disorder.
Health & Medicine
Using AI to predict hospital costs in real time
The extent of overdiagnosis is difficult to quantify but one report has suggested that an estimated 18-24 per cent of all cancers in Australia may be cases of overdiagnosis.
One reason behind overdiagnosis is the constant advance of technology, with newer, more sensitive tests being developed. Among the latest advances in medicine is the possibility of using the massive amount of data we generate every day to find clues that might point to undiagnosed diseases.
Throughout our day we use smartphones, type emails, interact on social media and count our steps on our smartwatches. Using artificial intelligence, the data can be used to find patterns that might help us detect diseases at an earlier stage.
The collection and use of medical data raise ethical concerns, like those of privacy and data security. Nonetheless, this is a very exciting time, and many benefits will probably emerge from these technologies. But just as with traditional diagnostic methods, overdiagnosis is still a risk that should be addressed. So, what can we do?
In our recently published paper, we propose using data banks of longitudinal patient information – data collected over long periods of time – to analyse the historical trajectories of different diseases and identify those subgroups of patients who won’t be significantly affected, if at all, by a given disease.
This data-driven approach could be used to improve disease definitions considering patient trajectories and identify the clinical attributes that might in the future allow clinicians to more accurately distinguish a diagnosis from an overdiagnosis.
Sciences & Technology
Are robots the answer for aged care during pandemics?
In a pilot study we conducted using a US hospital database with hundreds of thousands of hospital admissions, we trained an artificial intelligence algorithm to predict which patients were likely to develop sepsis in the next hours – sepsis is a complication of an infection in which the body’s own immune response begins to damage tissues and organs.
We then looked at the patients flagged by the algorithm who actually went on to develop sepsis, based on official diagnostic criteria. Among them, we found that almost 5 per cent of them had trajectories that appeared to be identical to those who never went on to develop sepsis. This suggests that we need more work on defining what is and what isn’t sepsis.
The huge amounts of data that technology allows us to collect and analyse is an opportunity to better identify people early who need treatment before they become sick. But we also need to use big data to ensure we aren’t taking perfectly well people and turning them into sick patients.
Banner: Getty Images