How to find the truth on Twitter
With more people using social media as a primary source of information, how can we sort the truth from the lies?
On 29 October 2012, the day Hurricane Sandy hit New York City, a rogue tweeter posted: “BREAKING: Confirmed flooding on NYSE [New York Stock Exchange]. The trading floor is flooded under more than 3 feet of water,” a message that quickly spread to the mainstream news.
Only it was not true.
More recently, these kinds of hoaxes, often sent with the intention to mislead, have been labelled ‘fake news’. Journalists, authorities and ordinary social media users can struggle to sort real news from this stream of misinformation.
BREAKING: Confirmed flooding on NYSE. The trading floor is flooded under more than 3 feet of water.
— Comfortably Smug (@ComfortablySmug) October 30, 2012
Social media is becoming many people’s main source of information, so finding a way to assess what is genuine and what is fake is increasingly important.
We have developed a framework, published in PLOS One, that assesses whether an event reported in a tweet is likely to be a witness account or not, by assessing the evidence of whether the tweeter is on-the-ground at the event.
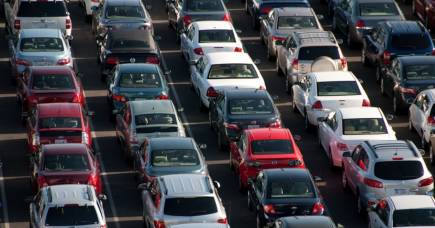
Ridesharing goes social
Assessing the trustworthiness of a tweet
Witness accounts are more trustworthy than hearsay – a principle long established in criminal proceedings. So, to assess whether a tweet is trustworthy, we need to decide whether it is reporting from first-hand experience.
Our framework, which builds on Marie Truelove’s earlier work, analyses details of a tweet to determine whether it is a witness account.
The most obvious starting point is the georeference in the metadata of some tweets, but only a small fraction of users turn this option on. To identify more sources of evidence we had to turn to the content of the tweet itself – the text and the pictures.
We look for inferences that the tweeter was at the event they are posting about, and then test that by seeking evidence they were not actually there at all.
In the text of the tweet, statements like observations of the event (like smoke in the sky for a bushfire), attached images (like a live shot from a football match), and the existence of geotags in the metadata all build a case for a user being a credible witness.
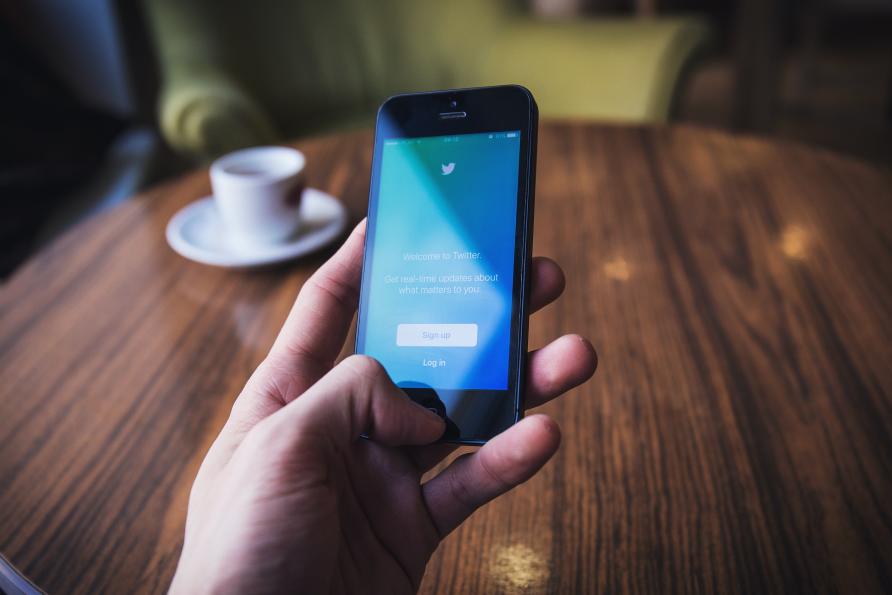
Further, we identify counter-evidence a tweeter is not a witness on-the-ground, for example if they describe themselves being in some other place or post an image of the event on a TV screen, and use this to test their evidence. If conflicting evidence is found their status can be investigated.
This evidence, which can be extracted automatically using machine learning, is then assessed to assign a tweet with a credibility measure, from low to high.
The challenges
Our framework has to overcome significant challenges, including assessing whether the tweet has been generated by direct experience of the event rather than watching it on TV.
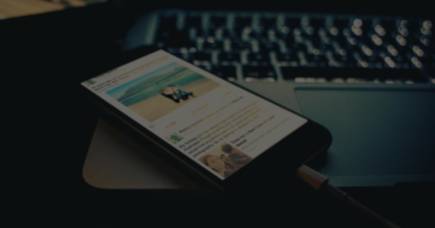
How gender shapes our Facebook chats
Attached pictures may be unattributed copies from other sources, or feature historic events at the same place. Tweeters can post their excited anticipation of attending an event later in the day but not go, or alternatively delay posting their witness accounts until on the way home after the event has ended.
Witness posting behaviour can also vary depending on the type of event. For example, tweets of anticipated attendance will not be detected unless an event is scheduled. And tweets reporting an event has not occurred will only appear if it was predicted in the first place, for example, when predicted flooding and power outages associated with a cyclone do not eventuate
We overcome these challenges primarily by investigating different evidence sources within tweets. A series of processes are applied to remove tweets that cannot support inferences the tweeter is at the event, for example, retweets.
Then we use supervised machine learning techniques to apply classification models to extract evidence from the remaining tweets that support inferences the tweeter is at the event.
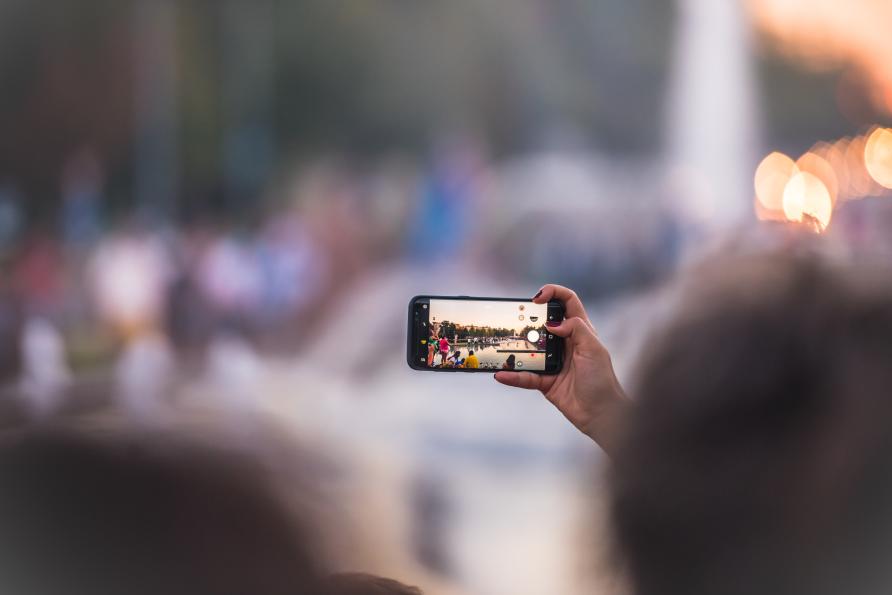
When multiple evidence is discovered for a single tweeter, it can be tested by combination, demonstrated using Dempster Shafer Theory of Evidence in the study published in PLOS One. This theory allows us to combine or fuse different types of evidence which support different levels of certainty.
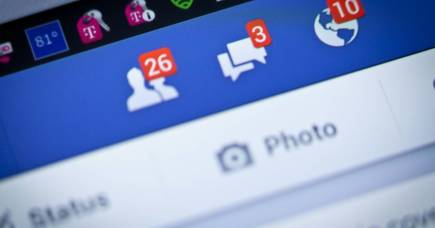
Facebook, the Government and revenge porn
checking the credibility
We found the inclusion of evidence from text and linked photographs can increase the number of tweeters identified at an event, in comparison to the number of tweeters identified from georeferences alone.
Additionally, the number of tweets that can be tested for evidence of corroboration or conflict increases when evidence and counter-evidence in their posting history is identified.
Using this framework, the tweet about the flooding of the New York Stock Exchange, would be assessed as having a low credibility measure, as there is a lack of evidence corroborating that the tweeter was on the ground, there was no picture providing further evidence, and the prior and next tweets are not sufficiently linked to the event.
If news organisations in particular had access to a framework like ours to assess these kinds of tweets purporting to be witness accounts, we could all trust our news a little more.
Banner image: Chris Slupski/Unsplash