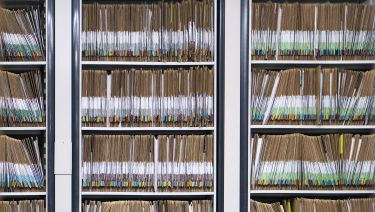
Sciences & Technology
The simple process of re-identifying patients in public health records
Last year, 15 million partially redacted public transport passenger details were posted online. It took researchers very little time to re-identify themselves and others, highlighting a risk to privacy
Published 15 August 2019
In mid 2018, Public Transport Victoria (PTV) released 15 million de-identified details of Melbourne’s contactless smart card ticketing system, known as Myki, to a data science competition – making them all available online.
Just three months later, in September, we had re-identified ourselves, a co-traveller and a member of the Victorian Parliament.
What’s concerning is that our analysis shows that most people in the released dataset are identifiable from just a handful of touch on or touch off events.
Information like this can then lead to further revelations like home and work locations; it can reveal regular patterns of travel; it can also tell us who card holders travel with, like family members or ex partners, or if they travel alone – like unaccompanied children returning from school often do.
This week, the Office of the Victorian Information Commissioner (OVIC) released their report on our re-identification.
Sciences & Technology
The simple process of re-identifying patients in public health records
The dataset was released in 2018 as part of a datathon – where teams of competitors analyse a real-world dataset.
More than 190 teams spent two months on the detailed information that PTV made available. It contained touch on and touch off events from the Myki ticketing system for over 15 million cards travelling between the middle of 2015 and the middle of 2018.
Nearly two billion rows of data were released, with the only de-identification being the redaction of the card IDs.
Each touch event included the exact time, to the second, along with the location of the event and information about the route travelled. While IDs were obscured, all trips on the same card were still linked.
The type of card was also listed, which may not initially seem like a bad thing, until you look into the 74 different card types.
Some card types can indicate sensitive elements, for example, a Federal Police Travel Pass, a Federal Parliamentarian Travel Pass or a State Parliamentarian Travel Pass.
In addition, there are card types for school children of different ages. Information presents both a security and safety risk by revealing the travel patterns for the card holder over a three-year period: when and where they travel, who they’re with and regular times when they’re traveling alone.
Sciences & Technology
Data privacy and power
Identifying ourselves was incredibly easy.
Knowing only two previous touch events from our online accounts allowed us to uniquely identify our records within the released dataset. Having found ourselves we were then able to analyse our own trip histories and perform a novel co-traveller analysis.
By analysing the cards that touched on within five seconds before or after we touched on, we were also able to create a ranking of cards that co-travelled with us the most.
Not surprisingly, we appeared towards the top of each other’s co-traveller rankings as a result of regularly making trips together to meetings in the Melbourne CBD.
But we could identify a child concession card that appeared high in one of our co-traveller rankings, which told us that there was a family connection.
After finding ourselves, we expanded our co-traveller analysis to evaluate whether it was possible to identify a targeted individual.
By finding a single occurrence of co-travelling with a colleague, it was possible to use our own auxiliary information to find the touch on event for that occurrence for our own card, and then analyse all the cards that touched on either five seconds before or after that time.
Politics & Society
What a second flaw in Switzerland’s sVote means for NSW’s iVote
This revealed a single card that could be a match to our colleague and further investigation of their travel history confirmed that it was them.
It’s not difficult to see how information like this could be used for nefarious purposes – for stalking by a jealous ex-partner, a rejected date, or something equally serious.
The longitudinal nature of this data means that re-identifying a card leads to the disclosure of substantial information about people’s travel behaviours, work and home locations and even, who they travel with.
Our investigation also demonstrated the ability to re-identify a person without co-travelling information.
We identified the card belonging to a state MP through nothing more than their tweets about travelling on public transport.
Most of us do not think twice about tweeting when our train or tram is late, without realising it reveals our time, location and route.
This information can allow for re-identification in datasets like this one and further highlights the risks of released unit record level data when large auxiliary datasets, like social media, are widely available.
Politics & Society
Is it really a myth that our data isn’t safe?
So, could this dataset have been safely released if further simple de-identification had been applied?
We examined the uniqueness of random sets of events for each card at different levels of timing precision, with and without the inclusion of location information.
Our analysis shows that even if times were reduced to the nearest five minutes, more than 50 per cent of the cards were uniquely identifiable after just five randomly selected touch on events.
With location included, it took only two randomly selected events.
To safely release a dataset, the organisers could have looked to differential privacy, a framework studied by us and numerous groups worldwide, and recently used by Transport for NSW in their 2017 Opal card data release.
Differential privacy is a mathematical security property that guarantees that attributes of any individual record are indistinguishable from a data release.
This most recent example of re-identification is not the first time a government has released unit record level data about the public in a claimed de-identified form that has then been re-identified fairly quickly.
The fallacy of de-identification is well known, but the same mistakes keep happening.
We need to make privacy the number one priority, above the desire to release and share data, to avoid further repeats of more mistakes like this one.
Banner: Paul Burston/University of Melbourne