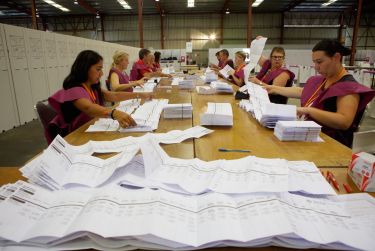
Sciences & Technology
Paper audits crucial for automated counting
Publishing data can bring benefits, but it also can be a great risk to privacy
Published 29 September 2016
Using only publicly available information, we have been able to decrypt the service provider ID numbers in the 10% sample of Medicare Benefits Schedule (MBS) published recently at the Federal Government’s data.gov.au website. We did not decrypt Patient ID numbers.
This research work is aimed at understanding mathematical facts about encryption and anonymization, in order to ensure that the security of government data is preserved in the face of the inevitable efforts of external parties who may be prepared to break the law and attempt to re-identify the data. There are numerous benefits to open government data, but it’s important to understand the mathematical techniques for protecting that data, so that the benefits can be derived with a clear understanding that individual privacy is not breached.
The department of health has made their own announcement about this work today. We notified them on 12 September, 2016 about the problem. The Department of Health then immediately removed the dataset from the website, opened lines of communication with us to further understand the issue and conducted their own careful investigation of the problem.
The MBS 10% sample dataset does not include names or addresses, but the implications of exposing individual provider IDs are still serious.
The encryption algorithm was described online at data.gov.au. That was the right thing to do, because it made it possible for us to identify weaknesses in the encryption method. Leaving out some of the algorithmic details didn’t keep the data secure – if we can reverse-engineer the details in a few days, then there is a risk that others could do so too.
Security through obscurity doesn’t work – keeping the algorithm secret wouldn’t have made the encryption secure, it just would have taken longer for security researchers to identify the problem. It is much better for such problems to be found and addressed than to remain unnoticed.
The MBS 10% sample was constructed by choosing a random 10% of Medicare patients. For each person selected, almost all Medicare claiming activities from 1984 to 2014 were included in the data. A separate dataset lists Pharmaceutical Benefits Schedule (PBS) claims for the same patients.
Most of the data are not encrypted, including Medicare item codes for tests and doctors’ visits, pharmaceutical benefits (PBS) item codes, and other information such as pricing and location. The codes describe what medical activity occurred, for example a particular kind of test, a particular prescription, or a visit to a GP or specialist. The ID numbers describing who received or provided the service are encrypted.
The dataset does not include names or addresses of patients or doctors – the MBS dataset includes an encrypted patient ID number and provider ID for each activity. Note that the patient’s ID number is not the number written on their Medicare card, and that the PBS dataset does not include the prescribing doctor’s ID.
The website states that: “A suite of confidentiality measures including encryption, perturbation and exclusion of rare events has been applied to safeguard personal health information and ensure that patients and providers cannot be re-identified.’’
Strictly speaking, the method of obscuring patient and provider ID numbers is not encryption, though we will use that term to refer to it for consistency.
Sciences & Technology
Paper audits crucial for automated counting
There are two main ways of trying to identify individuals in this kind of dataset:
Linkage attacks use the unencrypted data to identify people by linking the record with other known information; and
Cryptographic attacks reverse the encryption algorithm to recover encrypted data.
This article concentrates on successful cryptographic attacks.
Linkage attacks have been demonstrated on other datasets, for example location data, social network data and US health data, but we have not attempted them here.
Partial details about the linkable encryption algorithm were described online at data.gov.au, but were later removed at the same time as the dataset. Although neither the exact algorithm nor the details of subsequent processing were described in detail, we could guess those details for provider IDs and use the dataset to check our hypothesis. We were able to decrypt every service provider ID in the MBS dataset.
The details for patient ID numbers are different. We have not decrypted them.
Publishing data can bring great benefits to research but also great risks to privacy. The mathematical details matter: it’s a technically challenging task to understand whether a particular algorithm securely encrypts data or not. Datasets containing sensitive information about individuals clearly deserve more caution than others, and may not always be suitable for open public release.
The Australian Government’s open data program provides numerous benefits, allowing better decisions to be made based on evidence, careful analysis, and widespread access to accurate information.
Decisions about data publication itself should follow the same philosophy.
We have some important decisions to make about what personal data to publish and how it should be anonymised, encrypted or linked. Making good decisions requires accurate technical information about the security of the system and the secrecy of the data.
Details about the privacy protections should be published long in advance.
They can then be subject to empirical testing, scientific analysis, and open public review, before they are used on real data. Then we can make sound, evidence-based decisions about how to benefit from open data without sacrificing individual privacy.
Banner image: AAP Image/Joel Carrett
Since notifying the Department of Health of this issue, the authors have been in discussions about potentially being contracted to research the extent of the problem and possible mitigations and solutions for the future.