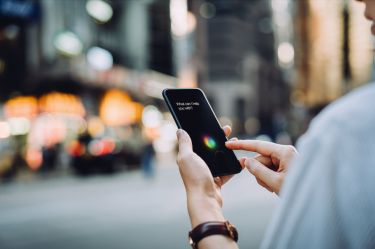
Politics & Society
AI and humans: collaboration rather than domination
Hospitals only know how much a patient costs once they’ve gone home, making it difficult to plan spending efficiently, but predictive algorithms can estimate costs soon after diagnosis
Published 13 July 2021
In many health systems around the world, running a hospital is a knife-edge balancing act.
On one side are the doctors and nurses providing urgent, even critical care to patients. Their job is to do all they can.
On the other side are the hospital administrators making sure there are enough resources – medicines, equipment, beds, staff – to cope. Their job is to ensure spending on patient care stays efficient so that the hospital can stay open.
But how much spending on a patient is enough?
In middle and high-income countries, hospitals are typically funded by government agencies and/or health insurance companies that reimburse them for their costs. But it isn’t a blank cheque.
Politics & Society
AI and humans: collaboration rather than domination
Each patient is retrospectively ‘coded’ to a particular patient group based on their condition, which is matched to an agreed estimate of what that patient would normally cost to treat. If the hospital has spent more than the coded amount then they lose money.
It is a system designed to keep hospitals efficient and is known globally as the diagnosis-related group (DRG) system. Originating in the US, versions of the DRG system operate in countries as diverse as Australia, China, Germany and Thailand.
But it has a huge drawback – all of this meticulous coding using hospital records and specialist assessors takes weeks or months. It means the cost of a patient’s care is only determined once the hospital has already used the resources and spent the money to keep that patient alive and well.
As a result, providers usually learn about service costs long after their patients have gone home.
This makes it difficult for a hospital to plan and allocate resources efficiently in real-time. It means hospitals are regularly missing opportunities to better plan day-to-day resource needs and manage high-risk (and high-cost) patients efficiently.
What is needed is a way to generate accurate estimates of costs early in a patient’s care. One way to do this is by using artificial intelligence.
Sciences & Technology
The danger of surveillance tech post COVID-19
In our recent research, we looked at whether we could build a computer model – an algorithm – to estimate a patient’s length of stay and costs based on the clinical notes written by doctors and nurses to document the course of the patient’s care.
The idea was to try and generate an accurate estimate of the cost that would assist a hospital in anticipating future resource requirements and planning at the point of care, instead of learning the information weeks later.
We applied a deep learning-based natural language processing (NLP) model on the clinical notes written by healthcare providers to classify a patient into an anticipated DRG code.
We focused on data from the first day of intensive care unit (ICU) admission because these first critical hours of care give a huge amount of important information.
Our experiments with a critical care data set called MIMIC-III from a large medical centre in the United States – paid under two DRG systems – demonstrated the potential of artificial intelligence to make early patient cost classification and estimate likely costs.
The data set includes 40,000 patients who were admitted to the critical care units of the Beth Israel Deaconess Medical Center in Boston, MA. The computer model was trained using routine clinical notes written by caregivers and specialists – like physician notes and radiology reports.
Sciences & Technology
Engineering COVID-19 protection
Once the model was trained we then evaluated it by testing it on new patient data.
The data set includes over 1,500 patients reimbursed through the US Medicare DRG, and over 2,000 patients reimbursed through a commercial DRG.
It turns out that it is challenging to precisely predict the discharge DRG for an individual patient using only limited data on a patient. However, by using notes data rather than only clinical measurements of vital signs and lab values, we were able to improve the results by as much as 30 per cent for the most common DRGs.
We also tested the computer model on the hospital’s so-called Case Mix Index (CMI), which simply averages the DRG payment weights of a group of patients and is often used by a hospital as an easier way to estimate overall costs.
The notes-based computer model showed promising performance on CMI prediction for cohorts of 500 patients under both DRG systems – the difference between the final actual CMI cost and that predicted by the model was less than 15 per cent.
This means it could help hospitals to forecast, instead of review, the needs of patients in their care and so better arrange resources to meet them.
The promise of big data to support managing high-risk patients and save costs in the high-spending healthcare industry is clear from the research. As is the value of applying big data approaches to clinical text data to provide clinical decision support, but its use in hospital operational planning had previously been unexplored.
Our work shows the feasibility of using artificial intelligence to help hospitals better allocate resources and meet the needs of patients. We hope our findings will be of interest to the communities that work towards utilising big data to promote efficiency and quality in healthcare.
Banner: Getty Images
We acknowledge Aboriginal and Torres Strait Islander people as the Traditional Owners of the unceded lands on which we work, learn and live. We pay respect to Elders past, present and future, and acknowledge the importance of Indigenous knowledge in the Academy.
Read about our Indigenous priorities