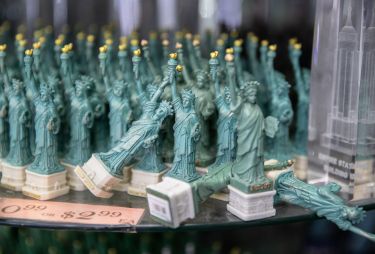
Sciences & Technology
When tools for a health emergency become tools of oppression
The UK’s misguided attempt to use algorithms to estimate school scores is a warning and reminder of the need to keep humans and accountability in automated decision-making
Published 15 September 2020
Researchers and journalists alike have taken great delight in recounting the new chant that student protests have used in the streets of the UK last month.
“F*** the algorithm, f*** the algorithm.”
The students were protesting against the algorithm that took their pre-COVID grades and spat out their final A-level results.
The algorithm, developed by UK exam regulator, the Office of Qualifications and Examinations Regulation (Ofqual), used four factors, which included the schools’ historic grade distributions. Unsurprisingly, students from historically low performing schools were disproportionately affected by the algorithm, with many concerned for their entrance into university.
Private schools saw a 4.7 per cent increase in grades above an A from last year, whereas government funded schools saw an increase of only 2 per cent.
Sciences & Technology
When tools for a health emergency become tools of oppression
Ultimately, the UK government backed down and students’ results will now be based on the grades that were predicted by teachers based on their students’ progress. However, this is the latest disturbing precedent of how governments’ careless use of algorithmic decision-making can have devastating consequences for citizens and particular social groups.
Algorithms, in their most utopian sense, could ensure that decision-making is more efficient, fair and consistent. Algorithms could, if designed and regulated with ethical considerations in mind, make decisions more objective and less biased. But this isn’t as straightforward as it seems at first glance.
While it is comforting to believe data can be relied on because it is neutral, in actual fact data isn’t neutral.
Using historical data to predict results can be discriminatory. In the case of the Ofqual debacle, schools exist in geographical spaces, so the students who go to them typically fall into the class and race groups of the area. By taking into account schools’ historic grade distributions, the Ofqual algorithm effectively penalises high performing students in low performing schools.
This creates artificial barriers for students in low performing schools, which are often those situated in lower socio-economic areas.
When it comes to people, predictive algorithms don’t account for a multitude of factors that might determine a person’s success. Perhaps a student did poorly in their practice test, but went on to study diligently for their final test, receiving much better results? What if the initial test was simply taken on a bad day?
Sciences & Technology
The privacy paradox: Why we let ourselves be monitored
Digital Ethics generally relies on the principles of transparency, fairness, and accountability. In terms of transparency, those using algorithms should be able to explain how the algorithm works, what data is being used, and how this will provide an outcome. The algorithm should be fair. Overtly biased data should be excluded and measures should be taken to mitigate where bias could arise from the data that is included.
Accountability ensures that there is a responsible party – a government department or a business cannot deploy an algorithm and absolve themselves of responsibility from the outcome.
But accountability also means ensuring that people can contest the decisions being made using algorithms. Contestability is the principle that algorithms can be challenged. In the same way you might dispute a parking fine or make a complaint about a product, you should also be able to raise a complaint or contest the outcome of an algorithm.
In relation to Ofqual’s algorithm, it was entirely predictable that at least some students would be unhappy with their results and would wish to contest their grades. Yet, before final grades were released there was no agreement on what the appeals process would be – initial guidance was released by Ofqual and then quickly revoked.
What would the students be able to appeal? The use of the algorithm? Their ranking? The use of their school’s historical data?
Another important aspect is who would students be able to appeal to, and how long would the appeal take? Even if their grades were later adjusted upwards, many students could have lost their conditional offers at universities.
Politics & Society
AI: It’s time for the law to respond
Ensuring there is a process for challenging the outcome before deployment is a key step towards keeping algorithmic decision making in check.
Finally, the decision to have the Ofqual algorithm as the sole decision-maker was a problem. Humans and machines are capable of working together, so why do we continue to build decision-making tools as if they don’t?
We can readily build processes in which algorithms streamline decisions while integrating these decisions with human-based decisions; the machine can process large amounts of data and the human can provide context and insight. For example, there should be a process for teachers to weigh in on the progress of their students, while scope can be given for lawyers or philosophers to consider the fairness and the ethics of algorithm-driven decisions.
Protections and appeal processes need to become common practice when algorithmic decision-making is used. Unless we start building fairer, transparent and explainable algorithms with appeals processes this will not be the last time we hear “f*** the algorithm” ringing through the streets.
Banner: Students in London protesting the UK government’s later aborted plan to change to how their final school scores are estimated. Getty Images