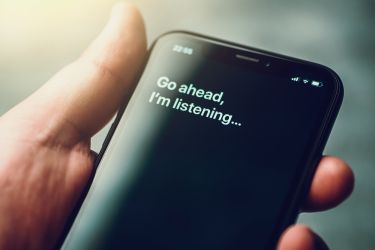
Sciences & Technology
The AI pretenders
While debate over the alleged sentience of the LaMDA chatbot continues, there are bigger questions about AI’s overall lack of transparency
Published 16 June 2022
The recent claims that Google’s chatbot LamDA had an existential discussion with a senior software engineer has grabbed the world’s attention.
Google employee Blake Lemoine claimed that talking with LaMDA is like talking to an “8-year-old kid that happens to know physics”. Lemoine is convinced that LaMDA is a sentient, human-like intelligence.
This isn’t the first time a chatbot has caused such a dramatic debate.
The first ever chatbot, created in 1966 by computer scientist Professor Joseph Weizenbaum at Massachusetts Institute of Technology (MIT), was comparatively simple by today’s standards. But to users like Professor Weizenbaum’s secretary, the bot, named ELIZA, seemed to “understand” them.
Almost 60 years later, chatbots are now widely used.
Sciences & Technology
The AI pretenders
You may have encountered them in communicating online with telecommunication companies, banks, airlines and shopping sites. They are usually found in a little box-shaped icon that pops up in the lower right-hand corner of the screen, asking you to type a question. And some of the answers can feel quite human.
So how do these chatbots engage with us in human-like ways?
The technology underpinning chatbots has evolved since the 1960s. The seminal advancement was the invention of data-driven algorithms – otherwise known as machine learning algorithms or artificial intelligence (AI). AI enables the kind of ‘conversations’ that LamDA has had with humans.
Chatbots are essentially computer programs that interpret written or spoken language and provide appropriate responses. Many programming languages and technologies can be used to build them. Regardless of approach, chatbots are of two basic types: ‘rules-based’ chatbots, like ELIZA, and ‘smart’ chatbots, like LaMDA.
Rules-based chatbots are the simplest kind. They are easy to build, predictable in output and relatively simple to maintain. This makes them a good choice for many practical purposes, like online banking.
Rules-based chatbots detect certain words or word combinations and pattern match them to a response or class of responses. They do this by following a pre-determined rule.
Sciences & Technology
TikTok captures your face
For example, the program may detect the word ‘hello’ in the user’s input “Hello there!” and match it to an appropriate greeting like, “Hi, my name is ChatBot!”.
The difficulty is that the range of possible inputs (things that can be said to or asked of the chatbot) is practically infinite. Any sequence of letters can be used to address the chatbot, but the chatbot can only respond to patterns that it ‘recognises’ with a corresponding ‘rule’.
And this is the key disadvantage of the simpler pattern matching chatbots.
‘Smart’ chatbots using more advanced techniques can overcome this limitation. They employ machine learning algorithms that perform Natural Language Processing (NLP). The complex type of algorithm they typically employ is a ‘neural network’.
A ‘neural network’ is a type of algorithm that has connections that are very roughly like biological neurons. The neural network must be ‘trained’ on sample data – meaning the algorithm iteratively compares its predictions to what the ‘correct’ output should be and then performs complex calculations, adjusting its parameters to improve its predictions over time.
The AI in smart chatbots is still performing pattern recognition, but unlike simple rules-based chatbots that rely on pre-programmed instructions, smart chatbots decipher the ‘rules’ or ‘patterns’ for themselves.
It means that smart chatbots can respond to a wider range of inputs. But it also means that this flexibility can be accompanied by greater unpredictability and less control.
Sciences & Technology
Challenging decisions made by algorithm
A now infamous example of this problem was Microsoft’s chatbot Tay. Tay learned its responses from human posts on Twitter. In less than 24 hours, Tay began mimicking Twitter users with its own racist and antisemitic statements.
Microsoft quickly shut it down.
This example highlights the difficulty operators face when it comes to controlling chatbots with AI neural networks. They are far less predictable and harder to maintain.
This is why chatbots in customer service roles currently use simple, pre-determined rules which are sufficient for most purposes.
All of this however doesn’t mean customer service chatbots are free from risk.
We are currently analysing our findings in a project funded by the Australian Communications Consumers Action Network on the use of chatbots providing customer support in Australian telcos.
Some of our concerns are around an overall lack of transparency when it comes chatbot use – as opposed to a problem of sentience.
Particularly, service chatbots may introduce themselves as a ‘virtual bot’, but if consumers don’t know what a virtual bot is they won’t know when they are talking to AI as opposed to a person.
Politics & Society
What is the law when AI makes the ‘decisions’?
This is problematic because some research suggests that this incorrect assumption makes consumers more trusting of the bot.
Additionally, most service chatbots don’t tell consumers what happens to the transcript of the conversation afterwards – raising further privacy concerns. And it’s simply unclear whether service chatbots are accessible or equitable in who can use them.
These pressing privacy concerns aside, ultimately, all chatbots are just computer programs designed by humans and used to interpret and respond to written language.
Understanding how chatbots work means we can better see their benefits and limitations. They can both be very useful as well as producing unpredictable or even offensive responses.
Chatbots, even the simpler ones like ELIZA, can respond in a way that is uncannily human. Smarter bots with very sophisticated neural networks take this to the next level.
But even smart chatbots like LamDA are just performing pattern recognition. So, we can exercise a healthy scepticism about claims that chatbots are sentient or virtually human.
That said, these discussion about the sentience of a chatbot or an AI are good debates to have – partly because the issue causes such confusion and partly because it helps us to reflect on what it is to be human – not because Lemoine may be right about LamDA or some future AI.
More importantly, it’s worth pausing to reflect on whether service chatbots are transparent, fair and effective for everyone – particularly when used as support for essential services like telecommunications.
Banner: Getty Images