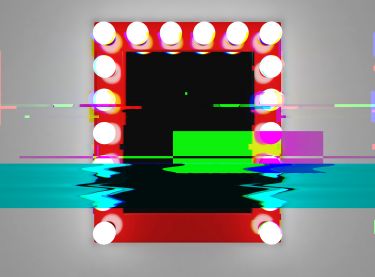
Politics & Society
Holding a black mirror up to artificial intelligence
Combatting bias and creating more inclusive AI is unlikely to succeed unless developers include those people who have been historically excluded or ignored
Published 24 October 2018
Advances in automation, machine learning and artificial intelligence (AI) mean we’re on the threshold of discoveries that could change human society irreversibly – for better or worse.
From AI that sorts CVs and shortlists job applicants, to facial recognition algorithms, these technologies are bringing new efficiencies and reducing errors in both public and private sector decision making.
But, increasingly, many of us are becoming aware of the dangers of bias and discrimination inherent in these technologies. A recent MIT study measuring how the technology works on people of different races and gender found that facial recognition AI was less accurate when classifying the faces of people with darker skin.
Meanwhile, Amazon scrapped an AI recruitment tool after the company found that it wasn’t rating candidates for software developer jobs and other technical posts in a gender-neutral way.
Some of these issues and concerns have already been raised by people like Cathy O’Neil, a data scientist who wrote the bestselling Weapons of Math Destruction, and political scientist Virginia Eubanks in Automating Inequality.
Politics & Society
Holding a black mirror up to artificial intelligence
But there are several reasons why AI may produce decisions that are tainted by bias or discrimination – and they have serious implications for those who develop and work with AI.
Chief among the concerns about discriminatory AI is the quality and scope of the data used to inform the automated process. AI ‘learns’ by referring to the data that humans ‘feed’ it. If certain groups of people are left out of the data set, the automated process won’t capture their characteristics.
Amazon’s now-abandoned recruitment tool is just one example of this. The data used to train the computer model to select the ‘right’ person for the job was drawn from the resumes of employees that had previously been selected for positions – most of them were men, reflecting male dominance across the tech industry.
So the system was trained to select against women and the types of educational and workplace experiences more common to women.
But these problems with data quality and inclusiveness are also exacerbated by the fact that AI is often a ‘black box’ – meaning the content and how it is used to inform decisions isn’t transparent.
Another reason why AI might produce biased decisions relates to what AI is asked to do.
Programmers and developers sometimes make mistakes or ask the wrong questions.
The University of Melbourne’s Professor Bernadette McSherry, who specialises in mental health law and criminal law, has highlighted some of the difficulties in trying to map symptoms of mental health conditions via social media posts.
Other research has pointed out the fundamental flaws in the equation used by Centrelink to match their data with records from the Australian Taxation Office and issue ‘robo-debt’ notices to people allegedly overpaid social security benefit.
Sciences & Technology
What were you thinking?
The end result is an ongoing investigation into the “deeply flawed” program, that sent out 20,000 debt recovery notices to people who were later found to owe less or even nothing.
It’s also necessary to factor in a lack of scrutiny or clear evaluation of the results AI produces into understanding why the technology comes up with biased conclusions. Decisions with social, economic and/or political implications shouldn’t only be based on data-mapping trends and probabilities – they must also incorporate our values and our vision for the type of society we want to live in.
You may or may not have heard of dynamic pricing. It uses data to predict how much consumers are prepared to pay for items in online markets. This isn’t just supply-and-demand, it uses a customer’s data trail to predict what they’re willing to pay.
While this may be accurate, as a society, we may ask ourselves whether it’s right that some necessary products and services are effectively more expensive for some – or is it price discrimination?
There must be legal responses to address some of these concerns about AI bias by demanding:
Sciences & Technology
Rise of the robots
Transparency, in terms of what data is used and how it is used
Explainability: understanding and explaining why the automated system made the decisions in question
Strong data rights to control data collection and sharing
Accountability, which includes a right to challenge automated decisions and seek redress for wrongful results, and a regulator with monitoring, investigation and enforcement powers.
Tech giants claim they are responding to community concern about algorithmic bias and are developing new ways to overcome hidden discrimination in order to support diversity in the outcomes of automated decision-making tools.
But efforts to combat bias and create more inclusive AI are unlikely to succeed unless developers adopt principles of universal design. This means including excluded or ignored communities in development, testing and evaluation processes.
Universal design is defined as an approach to “designing products, environments, programmes and services, so they can be used by all people, to the greatest extent possible, without the need for specialised or adapted features”.
As Dr Sheryl Burgstahler, the director of the University of Washington’s Accessible Technology Centre, explains – universal design requires the early identification of all those who will use or be affected by a device, algorithm, program, policy or law, and active involvement of those people in all stages of design, implementation and evaluation.
Key issues like access and inclusion must be repositioned as central aspects of the process, rather than ‘nice to have’ add-ons.
Sciences & Technology
When computers make art
The universal design processes require ongoing flexibility and responsiveness as well as training and education of all stakeholders to ensure that users’ involvement is meaningful and, importantly, is fed back into the design.
This approach is relevant not only to private tech companies, but is crucial to government and regulators in order to protect and advance the rights of all people.
Essentially, it means ensuring that data-sets are representative and, fundamentally, give people a choice and a voice about how their data is used.
A universal design process would likely avoid the most challenging of these issues.
Banner image: Shutterstock